About Google AI Research 2023 Report
Today, Google released an update about their progress in Artificial Intelligence. I am sharing some interesting highlights from the update in the following fields:
Transformer Computer Vision
Google reported progress in computer vision, specifically in the use of Transformer architecture in computer vision models. This approach, known as Vision Transformer, utilizes both local and non-local information in the image, which is more flexible than the localized feature-building abstraction of convolutions. However, this method has a high computational cost when applied to higher resolution images. In a new approach called "MaxViT", they combined local and non-local information while reducing computational cost, resulting in better performance on image classification and object detection tasks.
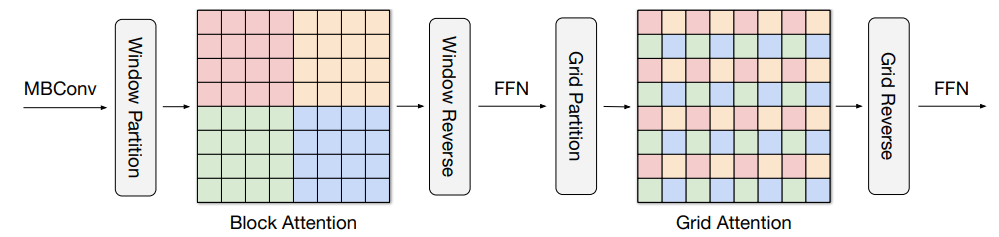
3D Generative
Google is making progress in understanding 3-D structure of real-world objects from 2-D images. They have been experimenting with different approaches such as "Large Motion Frame Interpolation" which creates slow-motion videos from two pictures taken seconds apart, even with significant movement in the scene. Another approach, "View Synthesis with Transformers," combines two techniques, "light field neural rendering" and "generalizable patch-based neural rendering," to synthesize novel views of a scene, which is a long-standing challenge in computer vision. These techniques enable high-quality view synthesis from just a couple of images of the scene.
Multimodal Models Transformers
Previous ML work mainly focused on models that handle a single type of data, such as language, image or speech. However, the future of ML lies in multi-modal models that can handle multiple types of data simultaneously. Google has been working on this in various ways, including "Multi-modal Bottleneck Transformers" and "Attention Bottlenecks for Multimodal Fusion" which uses a bottleneck layer to combine modalities after a few layers of modality-specific processing, resulting in improved accuracy on video classification tasks. This approach has also been applied to single-modality tasks, such as "DeViSE" which combines image and word-embedding representations to improve image classification, and "Locked-image Tuning (LiT)" which adds language understanding to pre-trained image models for better zero-shot image classification performance.
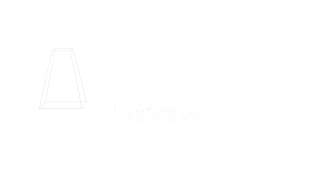
Generative Video
Google is working on creating generative models for video that can produce high-quality, temporally consistent videos with high controllability. This is a difficult task because, unlike images, videos have the added dimension of time. To meet this challenge, Google has made progress with two efforts, Imagen Video and Phenaki, which use different approaches. Imagen Video generates high-resolution videos using Cascaded Diffusion Models, starting with an input text prompt which is encoded into textual embeddings and then using a base video diffusion model to generate a rough sketch video, followed by multiple temporal and spatial super-resolution models to upsample and generate a final high-definition video. The resulting videos are high-resolution, spatially and temporally consistent, but currently only around 5 seconds long.
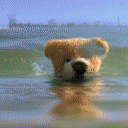
seo.call-to-action.title
seo.call-to-action.money-back
seo.call-to-action.message